From Countless Variables to a
Unique DNA Fingerprint
A Step Towards Personalised Medicine
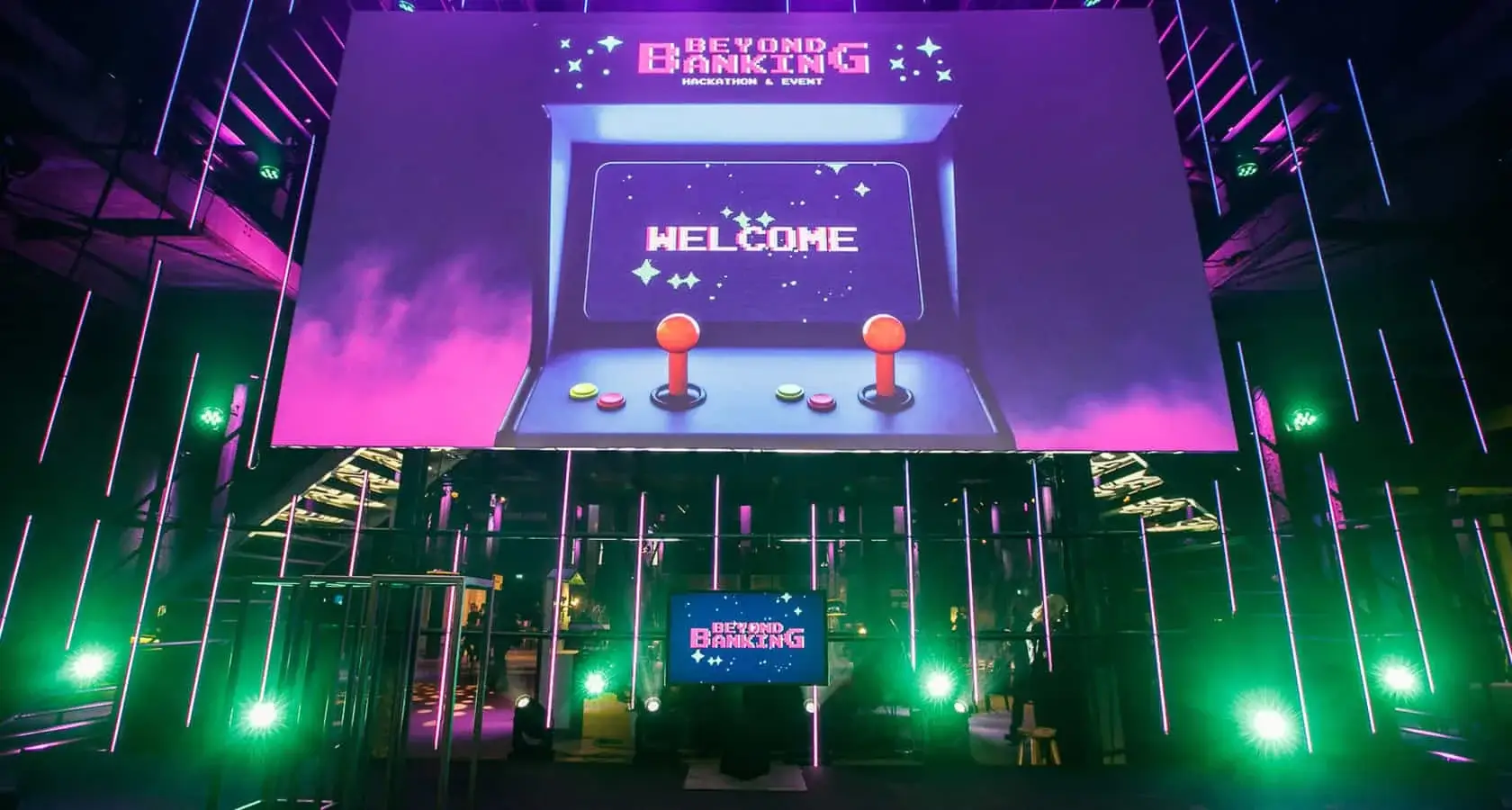
Date: 08-2018
SAS & Finaps contribute to cancer research at the Beyond Banking Hackathon
Team: Joost Huiskens, Rik de Ruiter, Turan Bulmus, Michael van Elk and Joran Roor
Article by Michael van Elk & Joran Roor
Beyond Banking Hackathon
On June 8th, 9th and 10th ABN-AMRO organised the 48-hour Beyond Banking hackathon at Sugar City in Halfweg. There were eight challenges on various topics including a healthcare case, which came about in collaboration with Erasmus MC and UMC Groningen. They were curious as to whether data scientists would be able to gain new insights from a patient’s genetic data, with the aim of better understanding cancer and better matching treatments with the patient’s genetic profile.
Both SAS and Finaps are already actively involved in the application of advanced analytics in healthcare, with projects such as the CAESAR-Project and Big Data for Small Babies. SAS and Finaps enthusiastically took the opportunity to contribute to cancer research, and formed a team that participated in this case.
Accelerating Cancer Research
Research into treatments for cancer is currently done by looking at a group of patients in order to determine which treatment is effective and which is not. However, we know that both the genetic makeup of the patient and the specific mutations in the tumor can have a major influence on the effectiveness of a therapy. Ideally, one would like to include this information in the consideration between different treatments.
Due to advances in technology to acquire genetic information from a patient, this information is becoming cheaper and easier to obtain. The hope is that these data not only give us more insight into the molecular basis of the disease, but also help us to determine the best treatment for the individual patient. The ideal image is a new era of personalised care, where everyone gets a tailor-made treatment.
There are many different types of genetic information that we can obtain. The most well-known of these concerns the DNA sequence, also called genomics. Additionally, there is epigenomics (a form of regulation of DNA expression), transcriptomics (the actual expression of DNA, measured in several ways), and proteomics (the final expression of proteins).
Because of the large amount of information that can be obtained per patient in this way, it turns out to be a great challenge for doctors and medical researchers to work with these datasets or draw conclusions from them. The medical world therefore has a great need for data scientists who can analyse this data in an integrated way to gain new insights.
Research questions
Several research questions were formulated for the Healthcare Challenge. These served as guidelines, it was up to the teams to come up with an approach that would answer one or more of these questions as well as possible. These questions were:
- Is it possible to visualise the correlations between the different datasets?
- Is it possible to divide patients into subtypes based on genetic information?
- Is it possible to estimate survival based on genetic information?
- Is it possible to predict the response to therapy based on the genetic information?
Approach
The following approach was formulated for solving these problems and answering the research questions:
- Loading, cleaning and merging the datasets.
- Reducing the number of variables to arrive at a personal genetic ‘fingerprint’ for each patient.
- Searching for subtypes based on this fingerprint.
- Investigate whether these subtypes differ in survival or response to chemotherapy.
Technical Details
Datasets
Seven datasets on lung cancer patients were made available: six with genetic information, and one with clinical data. The genetic datasets consisted of data on mutations, copy number variation, methylation, micro-RNA expression, messenger RNA expression and protein expression.
All of this for more than 1,000 patients, about half of whom had the subtype non-small-cell lung cancer, and half had the subtype squamous lung cancer. These subtypes are very different and have therefore been individually analysed. The analysis and results below concern the non-small-cell variant.
Merging the Data
Not every patient appears in every dataset. That is why a selection was made of the available tables to maximise the overlap of available patients. Based on this criterion, the micro-RNA and protein expression data were dropped, since they were available for far fewer patients. If there were multiple measurements for one patient within one table, these are aggregated by an average or transposition so that for each patient one row of measurements remains, with approximately 140,000 variables.
Data Preparation
This merged table still contained many missing values, so to create a complete table, a number of steps are performed. The variables containing more than 20% missing values are dropped, after which around 60,000 variables remain. The remaining missing values are estimated using a K-Nearest Neighbour algorithm, which determines the values by looking at patients with similar properties to which the relevant variable is known.
Because the algorithms applied to this data are sensitive to the scale of the values, all variables are standardised so that they have the same mean and the same variance. This prevents the variables with a relatively large scale from being unfairly highly weighted in the training process.
Dimensionality Reduction
The next challenge is the number of variables. 60,000 variables for 577 patients will undoubtedly lead to overfitting, because with so many of dimensions, spurious mathematical relationships can always be found in the training data set which will not generalise to other data. Within data science there are several methods to deal with this, and it depends on the situation which method is most suitable.
Cancer is known to be a consequence of mutations in the genome, and the resulting changes in the processes of the cell. The available datasets provide an overview of these processes at multiple points. Given the high complexity of the processes involved, it does not seem sufficient to investigate individual effects of the variables, since the relevant patterns will consist of combinations of many variables.
This is an ideal situation for neural networks, which are ideally suited to modelling complex relationships. An auto-encoder is a type of neural network capable of “summarising” a large number of variables in a smaller number of variables. It does this by trying to summarise the supplied data in such a way, so that the original data can be reconstructed again with maximum fidelity. This summary of a patient’s genetic data is like a fingerprint, so it is referred to as the personal multi-omics fingerprint.
Clustering
Due to the smaller number of variables, the personal multi-omics fingerprint is a much more suitable basis for further analysis. We apply the popular K-means clustering method to this summary in order to group together patients with a similar genetic profile. We can then further analyse the groups that arise from this, to see how they differ from each other. The first step in this is to see if there is a different survival for these groups; the results of this analysis come after these technical details. Analysis of the best treatment methods for different groups was also carried out during the hackathon, but unfortunately it turned out that the quality of the data related to treatment methods was inadequate for presentable results. Further analysis to examine for instance differences in genetic expression is possible, however, there was no time for this during the hackathon.
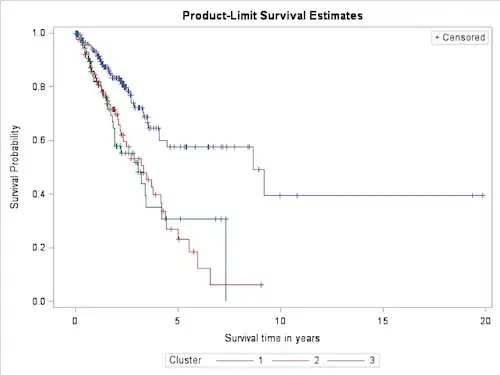
Preliminary results point to a distinctive method
Analysis of the clusters in the ‘Personal Multi-omics Fingerprint’ resulted in the Kaplan-Meier survival chart
It can clearly be seen that two groups can be distinguished that have a very different survival expectation. It is important to realise that this grouping is made using only genetic information, without considering clinical variables such as age, sex, stage of the tumor, etc. In fact, no significant difference is found between the clinical data of the groups. This means that this method of patient classification based on the personal multi-omics fingerprint was not possible on the basis of only the clinical data, which doctors currently base their prognosis on. This is a strong indication that this method can be of added value in clinical practice.
Next Steps
Despite not winning the hackathon, the results indicate a possible relevance for clinical practice. The results were presented to medical specialists and researchers who confirmed its potential. On the basis of this feedback we are currently working together on publishing these results in a scientific article.
Since the findings are the result of only one weekend research, there is still much work to do. Adjusting the settings of the auto-encoder can result in a better representation of the genetic information in the fingerprint, making the results more accurate. This can also be done by training the model for longer, or by adding more layers so that more complex relationships can be established. As is usual with medical datasets the number of patients is limited, so more data will clearly improve the reliability of the results. Other possible improvements include pre-training the network using genetic data from other sources, so that the network already starts with prior knowledge of common patterns. Finally, if higher quality data of treatments if available, the personal multi-omics fingerprint can be used as the basis for supervised classification models that predict the effects of various treatments.
Acknowledgements
The team is grateful for this opportunity to make a small contribution to cancer research. Special thanks go to the organizers of the hackathon, Tjebbe Tauber from ABN-AMRO, and all doctors and researchers from Erasmus MC and UMC Groningen:
Prof. Dr. Ing. Peter van der Spek (Erasmus MC Rotterdam)
Prof. Dr. Harry Groen (UMCG, Groningen)
Prof. Dr. Joachim Aerts (Erasmus MC Rotterdam)
Daan Hurkmans (Erasmus MC Rotterdam)
Menno Tamminga (UMC Groningen)
Rogier van Wijck (Erasmus MC Rotterdam)