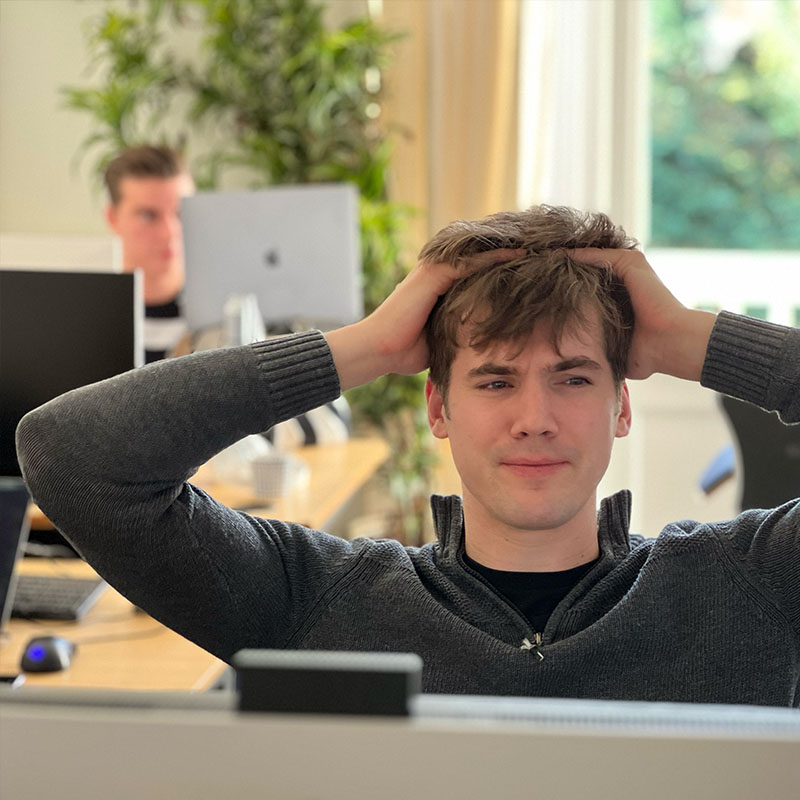
Getting data quality right
Rapid technological development has made it possible to use more and more data when making decisions. However, this data must be of high quality. Garbage in is garbage out. Only when the data quality is in order will valuable insights be gained from the analyses. To guarantee data quality, investments must be made in standardisation and validation; this requires awareness from the ground up. Data quality is as much a human as it is a technical challenge.
Lenard Koomen, Data Analytics Engineer, talks about his vision of data quality in this interview. How do you ensure high data quality from the ground up and how to start working on it?
In short
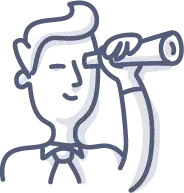
1. Lenards’ vision on data quality
Garbage in is garbage out
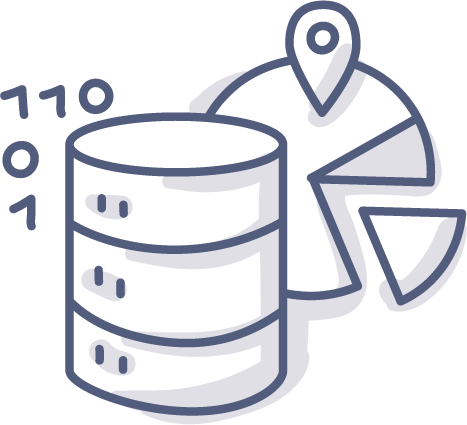
2. How to automate data cleansing
Data scientists spend 60-80% of their time preparing and refining data
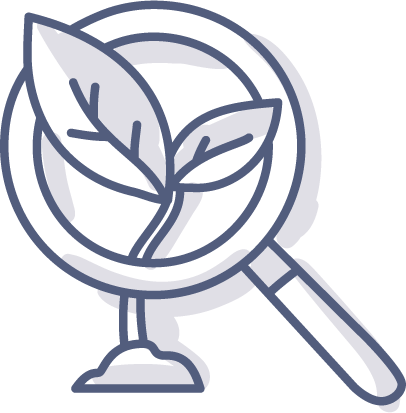
3. Prevention is better than cure
How to get started?
1. Lenards’ vision on data quality
Introducing
My name is Lenard, and during my Aerospace Engineering studies at TU Delft I became interested in machine learning, data science and AI. This interest eventually led to a job as a data analyst in the offshore wind industry, where I was responsible for monthly reports and integrating multiple external data sources into the system. Now I work at Finaps, as a Data Analytics Engineer, where I help clients make better use of their data.
“You don’t make things easier for a metal worker by handing over a container full of small different pieces of metal instead of a rectangular, homogeneous block of steel”
What is your vision on data quality?
I am particularly fascinated by the idea that there are insights hidden in a growing mountain of data. In a process similar to the mining and refining of iron ore, for example, the data is purified and processed, ultimately leading to a valuable end product.
The degree of purity of the raw material and the delivery of the right intermediate products are very important here; you don’t make things easier for a metal worker by handing over a container full of small different pieces of metal instead of a rectangular, homogeneous block of steel. I think this is obvious to a lot of people, but when it comes to data, people don’t seem to take it into account as much, with all the consequences that entails.
Ultimately, data quality is important because it is what people downstream depend on to make the right business choices. Ensuring data quality delivers value by reducing uncertainty and facilitating the setting of the right course for an organisation.
In my experience, poor data quality often arises at the start of the process, when data is gathered from different, often external, sources. The data is then initially packaged in a varied set of documents with various templates. Knowledge about the exact definition and nuances of each field usually does not survive this step and is hidden in the brains of the people involved in the process. Because data is often combined in complex ways, poor data quality can quickly spread through a process. An additional problem is that, unlike explicit programming errors, incorrect data cannot be easily spotted.
2. Getting started
How can you automate data cleansing?
There are various ways of cleaning up the data automatically and improving its quality. An example is correcting typos and spelling variations in place names. This can be done by comparing with a dataset of “correct” spellings, based on the processing distance. A second example is that missing data can be imputed, which means that it is filled in afterwards based on a data model. These methods make use of both specialist knowledge and the source data itself. What can you do with the time saved on data cleansing? However, polishing a dataset afterwards can cost a lot of time, knowledge and money. It is estimated that data scientists spend 60%-80% of their time preparing and refining data, something I personally underestimated when I started this work. As a result, organisation-wide improvements in data quality quickly generate value in the form of cost savings, more thorough analysis, clearer visualisation, and more motivated data analysts.
“It is estimated that data scientists spend 60%-80% of their time preparing and refining data.”
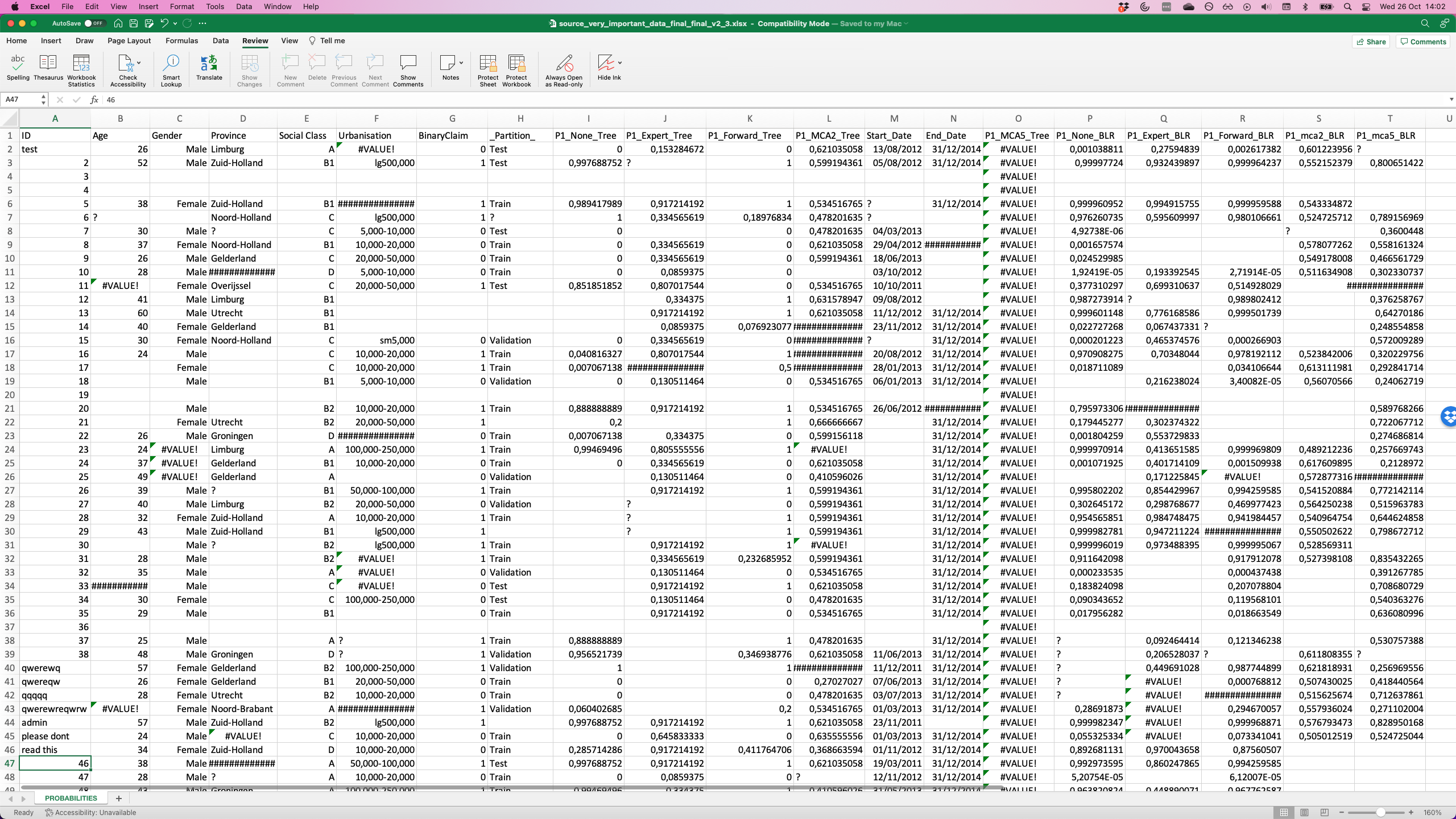
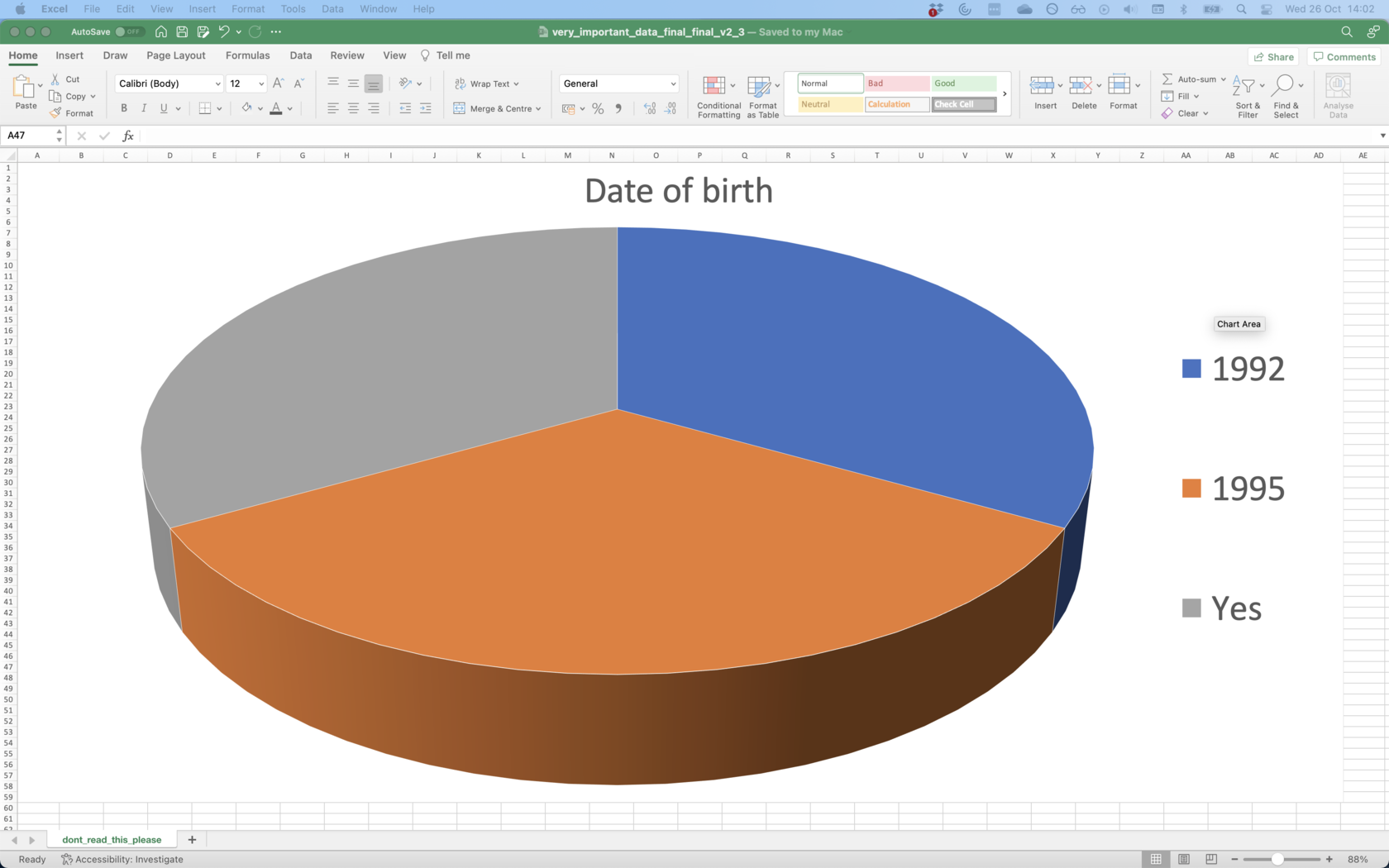
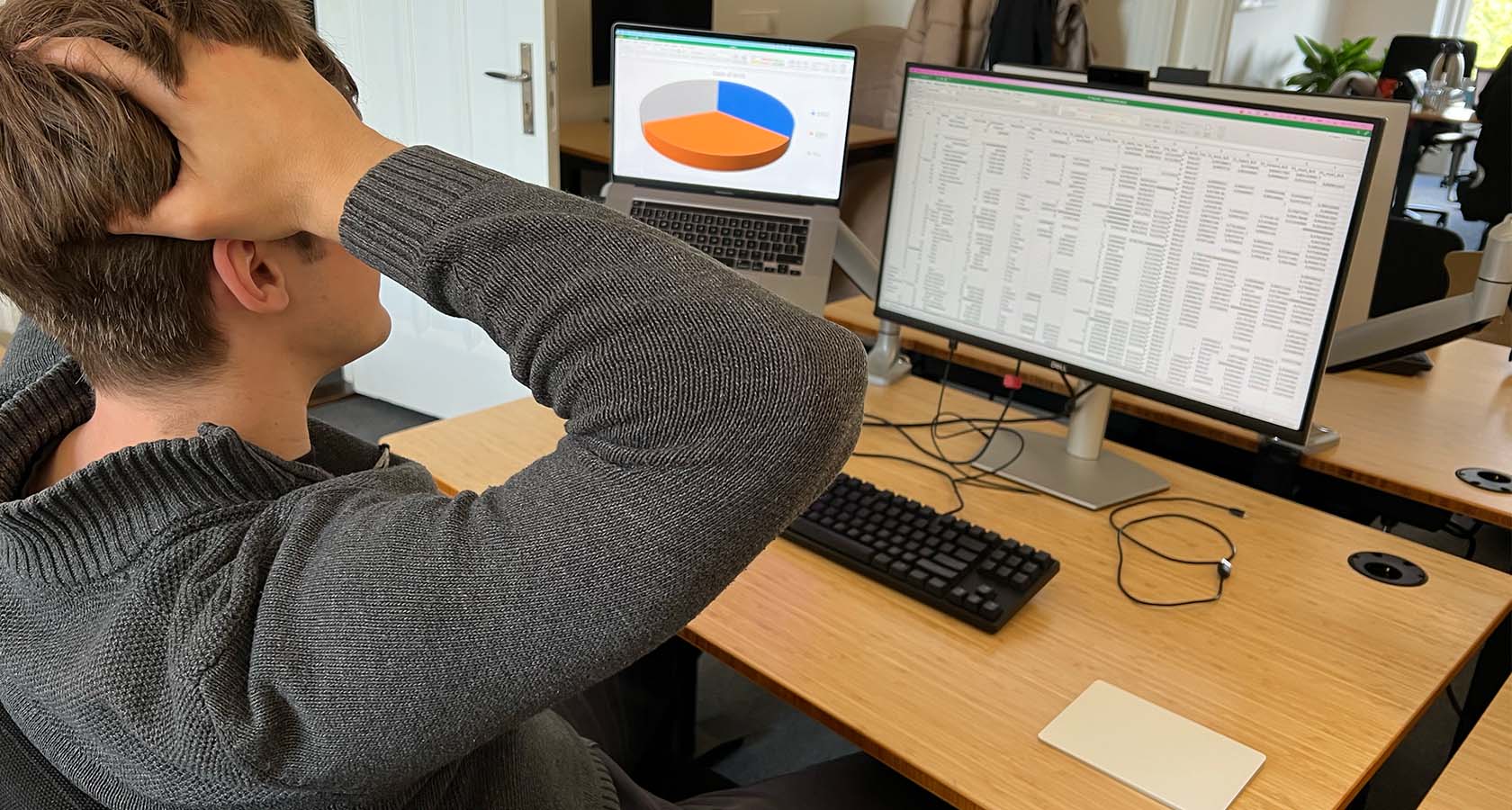
How do you ensure clean data from the ground up?
This is a typical case where “prevention is better than cure”, ensuring data quality is more effective and ultimately cheaper the closer it is to the source. Data must be validated immediately upon import. For this purpose, it is important that an organisation has a standardised data model on which validation can be based. With a standard data model, the data speak the same “language”, as it were, everywhere; this facilitates cooperation and makes it easier to maintain data consistency and quality. In order to form this model, it is important to sit down with stakeholders to create something that everyone supports, which goes hand in hand with making people more aware of the importance of data quality. This is complicated by the fact that the value generated by data is difficult to see at the point where it enters the organisation, while this is precisely the point where quality assurance is most effective.
“Prevention is better than cure”
3. Getting started
A practical first step towards improving data quality is to establish what this actually means in the context of your specific organisation. This requires drawing up clear rules and definitions, which together form the data model. This then forms the basis for mapping data quality within the process, by means of automated tests. The result is a clear framework within which data quality can be measured, discussed and improved.
What can we achieve in three months?
Become a more data-driven organization in three months. Extracting actionable insights from data, leading to better –more informed–decisions.
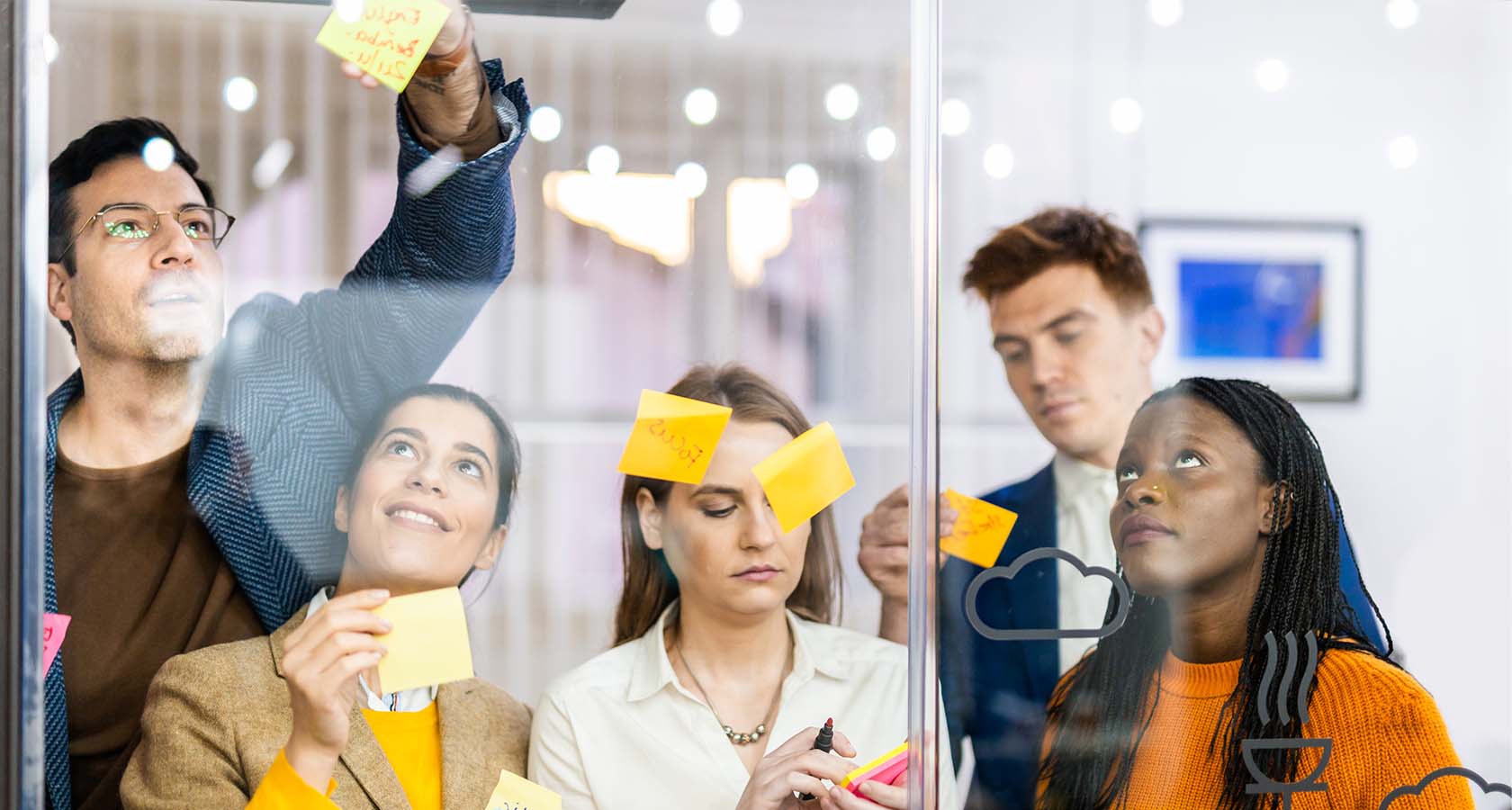
Getting started with the data kickstart service
1. Develop an MVP for a use case that helps the organisation in making a data-informed decision
2. Create a vision-document, including a roadmap and concrete action points for the coming period
3. Train employees’ data-literacy to embed all knowhow to be able to work with data within the organisation
Our cases
Our tech partners
Finaps uses fit-for-purpose (FFP) technologies, either Open Source or licensed. Mendix is our strong low-code platform partner, while we use SAS for its outstanding analytics platform. Our advice doesn’t depend on the available framework but is always based on the best possible solution for our client. As it should be.